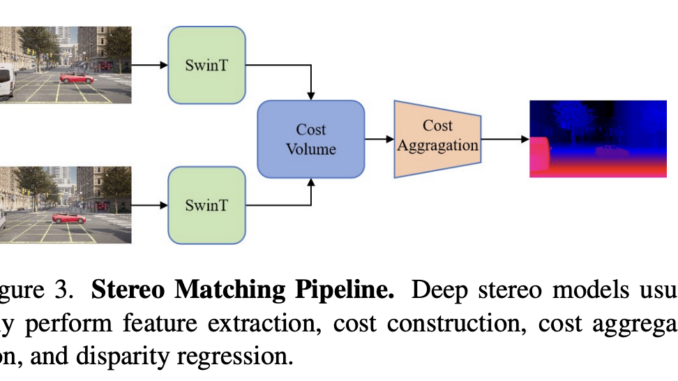
Computer vision is revolutionizing due to the development of foundation models in object recognition, image segmentation, and monocular depth estimation, showing strong zero- and few-shot performance across various downstream tasks. Stereo matching, which helps perceive depth and create 3D views of scenes, is crucial for fields like robotics, self-driving cars, and augmented reality. However, the exploration of foundation models in stereo matching remains limited due to the difficulty of obtaining accurate disparity ground truth (GT) data. Many stereo datasets exist, but using them effectively for training is difficult. Moreover, these annotated datasets cannot train an ideal foundation model even when combined.
Currently, Stereo-from-mono is a leading study focusing on creating stereo-image pairs and disparity maps directly from single images to address these challenges. However, this approach resulted in only 500,000 data samples, which is relatively low compared to the scale required to train robust foundation models effectively. While this effort represents an important step towards reducing the dependency on expensive stereo data collection, the generated dataset is still insufficient for building large-scale models capable of generalizing well to diverse real-world conditions. Early Stereo-matching methods mainly relied on hand-crafted features but shifted to CNN-based models like GCNet and PSMNet, improving accuracy with techniques like 3D cost aggregation. Video stereo matching uses temporal data for consistency but struggles with generalization. Cross-domain methods address this by learning domain-invariant features using techniques like unsupervised adaptation and contrastive learning, as seen in models like RAFT–Stereo and FormerStereo.
A group of researchers from School of Computer Science, Wuhan University, Institute of Artificial Intelligence and Robotics, Xi’an Jiaotong University, Waytous, University of Bologna, Rock Universe, Institute of Automation, Chinese Academy of Sciences and University of California, Berkeley conducted detailed research to overcome these issues and proposed StereoAnything, a foundational model for stereo matching developed to produce high-quality disparity estimates for any pair of matching stereo images, no matter how complex the scene or challenging the environmental conditions. It is designed to train a robust stereo network using large-scale mixed data. It mainly consists of four components: feature extraction, cost construction, cost aggregation, and disparity regression.
To improve generalization, Supervised stereo data was used without depth normalization, as stereo matching relies on scale information. The training began with a single dataset and combined top-ranked datasets to improve robustness. For single-image learning, monocular depth models predicted depth converted into disparity maps to generate realistic stereo pairs via forward warping. Occlusions and gaps were filled using textures from other images in the dataset.
The experiment showed the evaluation of the StereoAnything framework using OpenStereo and NMRF-Stereo baselines with Swin Transformer for feature extraction. Training used AdamW optimizer, OneCycleLR scheduling, and fine-tuning on labeled, mixed, and pseudo-labeled datasets with data augmentation. Testing on KITTI, Middlebury, ETH3D, and DrivingStereo showed StereoAnything significantly reduced errors, with NMRF-Stereo-SwinT lowering the mean error from 18.11 to 5.01. Fine-tuning StereoCarla on more diverse datasets lead to the best mean metric of 8.52%. This showed the importance of dataset diversity when concerning stereo-matching performance.
In terms of results, the StereoAnything showed strong robustness across various domains in both indoor and outdoor scenes. This approach constantly delivered a disparity map that was more accurate than with the NMRF-Stereo-SwinTmode. Thus, this approach shows strong generalization capabilities and performs better across domains with numerous visual and environmental differences.
It is safe to conclude that StereoAnything provided a highly useful solution for robust stereo matching. A new artificial dataset called StereoCarla is used to better generalize across different scenarios and enhance performance. Also, the effectiveness of labeled stereo datasets and pseudo stereo datasets generated using monocular depth estimation models was investigated. In terms of performance, StereoAnything achieved competitive performance across various benchmarks and real-world scenarios. These results show the potential of hybrid training strategies, including diverse data sources to enhance stereo model robustness, and can be used as the baseline for future improvement and research!
Check out the Paper and GitHub. All credit for this research goes to the researchers of this project. Also, don’t forget to follow us on Twitter and join our Telegram Channel and LinkedIn Group. If you like our work, you will love our newsletter.. Don’t Forget to join our 55k+ ML SubReddit.
🎙️ 🚨 ‘Evaluation of Large Language Model Vulnerabilities: A Comparative Analysis of Red Teaming Techniques’ Read the Full Report (Promoted)
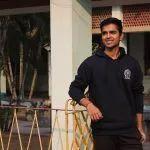
Divyesh is a consulting intern at Marktechpost. He is pursuing a BTech in Agricultural and Food Engineering from the Indian Institute of Technology, Kharagpur. He is a Data Science and Machine learning enthusiast who wants to integrate these leading technologies into the agricultural domain and solve challenges.
Be the first to comment