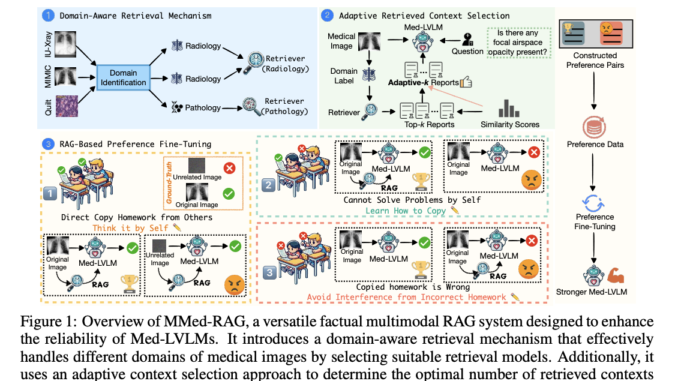
AI has significantly impacted healthcare, particularly in disease diagnosis and treatment planning. One area gaining attention is the development of Medical Large Vision-Language Models (Med-LVLMs), which combine visual and textual data for advanced diagnostic tools. These models have shown great potential for improving the analysis of complex medical images, offering interactive and intelligent responses that can assist doctors in clinical decision-making. However, as promising as these tools are, they are not without critical challenges that limit their widespread adoption in healthcare.
A significant issue faced by Med-LVLMs is the tendency to produce inaccurate or “hallucinated” medical information. These factual hallucinations can severely affect patient outcomes if models generate erroneous diagnoses or misinterpret medical images. The primary reasons for these issues are the need for large, high-quality labeled medical datasets and the distribution gaps between the data used to train these models and the data encountered in real-world clinical environments. This mismatch between training data and actual deployment data creates significant reliability concerns, making it difficult to trust these models in critical medical scenarios. Also, current solutions like fine-tuning and retrieval-augmented generation (RAG) techniques have limitations, especially when applied across diverse medical fields such as radiology, pathology, and ophthalmology.
Existing methods to improve the performance of Med-LVLMs primarily focus on two approaches: fine-tuning and RAG. Fine-tuning involves adjusting model parameters based on smaller, more specialized datasets to improve accuracy, but the limited availability of high-quality labeled data hampers this method. Also, fine-tuned models often need to perform better when applied to new, unseen data. Conversely, RAG allows models to retrieve external knowledge during the inference process, offering real-time references that could help improve factual accuracy. However, this technique could be even better. Current RAG-based systems often need help to generalize across different medical domains, which limits their reliability and causes potential misalignment between the retrieved information and the actual medical problem being addressed.
Researchers from UNC-Chapel Hill, Stanford University, Rutgers University, University of Washington, Brown University, and PloyU introduced a new system called MMed-RAG, a versatile multimodal retrieval-augmented generation system designed specifically for medical vision-language models. MMed-RAG aims to significantly improve the factual accuracy of Med-LVLMs by implementing a domain-aware retrieval mechanism. This mechanism can handle various medical image types, such as radiology, ophthalmology, and pathology, ensuring that the retrieval model is appropriate for the specific medical domain. The researchers also developed an adaptive context selection method that fine-tunes the number of retrieved contexts during inference, ensuring that the model uses only relevant and high-quality information. This adaptive selection helps avoid common pitfalls where models retrieve too much or too little data, potentially leading to inaccuracies.
The MMed-RAG system is built on three key components:
The domain-aware retrieval mechanism ensures the model retrieves domain-specific information that aligns closely with the input medical image. For example, radiology images would be paired with appropriate radiology-based information, while pathology images would be pulled from pathology-specific databases.
The adaptive context selection method improves the quality of the retrieved information by using similarity scores to filter out irrelevant or low-quality data. This dynamic approach ensures that the model only considers the most relevant contexts, reducing the risk of factual hallucination.
The RAG-based preference fine-tuning optimizes the model’s cross-modality alignment, ensuring that the retrieved information and the visual input are correctly aligned with the ground truth, thereby improving overall model reliability.
MMed-RAG was tested across five medical datasets, covering radiology, pathology, and ophthalmology, with outstanding results. The system achieved a 43.8% improvement in factual accuracy compared to previous Med-LVLMs, highlighting its capability to enhance diagnostic reliability. In medical question-answering tasks (VQA), MMed-RAG improved accuracy by 18.5%, and in medical report generation, it achieved a remarkable 69.1% improvement. These results demonstrate the system’s effectiveness in closed and open-ended tasks, where retrieved information is critical for accurate responses. Also, the preference fine-tuning technique used by MMed-RAG addresses cross-modality misalignment, a common issue in other Med-LVLMs, where models struggle to balance visual input with retrieved textual information.
Key takeaways from this research include:
MMed-RAG achieved a 43.8% increase in factual accuracy across five medical datasets.
The system improved medical VQA accuracy by 18.5% and medical report generation by 69.1%.
The domain-aware retrieval mechanism ensures that medical images are paired with the correct context, improving diagnostic accuracy.
Adaptive context selection helps reduce irrelevant data retrieval, increasing the reliability of the model’s output.
RAG-based preference fine-tuning effectively addresses misalignment between visual inputs and retrieved information, enhancing overall model performance.

In conclusion, MMed-RAG significantly advances medical vision-language models by addressing key challenges related to factual accuracy and model alignment. By incorporating domain-aware retrieval, adaptive context selection, and preference fine-tuning, the system improves the factual reliability of Med-LVLMs and enhances their generalizability across multiple medical domains. This system has shown substantial improvements in diagnostic accuracy and the quality of generated medical reports. These advancements position MMed-RAG as a crucial step forward in making AI-assisted medical diagnostics more reliable and trustworthy.
Check out the Paper and GitHub. All credit for this research goes to the researchers of this project. Also, don’t forget to follow us on Twitter and join our Telegram Channel and LinkedIn Group. If you like our work, you will love our newsletter.. Don’t Forget to join our 50k+ ML SubReddit.
[Upcoming Live Webinar- Oct 29, 2024] The Best Platform for Serving Fine-Tuned Models: Predibase Inference Engine (Promoted)
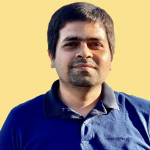
Asif Razzaq is the CEO of Marktechpost Media Inc.. As a visionary entrepreneur and engineer, Asif is committed to harnessing the potential of Artificial Intelligence for social good. His most recent endeavor is the launch of an Artificial Intelligence Media Platform, Marktechpost, which stands out for its in-depth coverage of machine learning and deep learning news that is both technically sound and easily understandable by a wide audience. The platform boasts of over 2 million monthly views, illustrating its popularity among audiences.
Be the first to comment