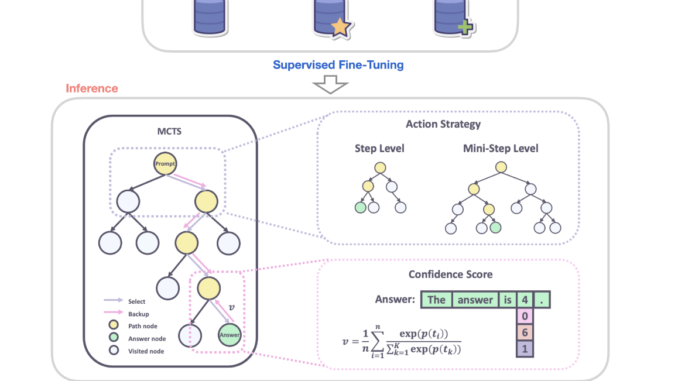
The field of AI is progressing rapidly, particularly in areas requiring deep reasoning capabilities. However, many existing large models are narrowly focused, excelling primarily in environments with clear, quantifiable outcomes such as mathematics, coding, or well-defined decision paths. This limitation becomes evident when models face real-world challenges, which often require open-ended reasoning and creative problem-solving. These tasks are difficult to evaluate because there are no universally accepted “right” answers or easily quantifiable rewards. The question arises: can an AI model be trained to navigate such ambiguity and still produce reliable results?
Alibaba Releases Marco-o1
Alibaba has released Marco-o1, a new AI model designed to advance open-ended problem-solving. Developed by Alibaba’s MarcoPolo team, Marco-o1 is a Large Reasoning Model (LRM) that builds on lessons from OpenAI’s o1 model. While the o1 model demonstrated strong reasoning capabilities on platforms like AIME and CodeForces, Marco-o1 aims to extend beyond structured challenges. The core goal for Marco-o1 is to generalize across multiple domains, especially those where strict evaluation metrics are unavailable. This is achieved by integrating techniques such as Chain-of-Thought (CoT) fine-tuning, Monte Carlo Tree Search (MCTS), and reasoning action strategies that enable Marco-o1 to handle complex problem-solving tasks more effectively.
Technical Details
Marco-o1 leverages several advanced AI techniques to enhance its reasoning capabilities. The model utilizes Chain-of-Thought (CoT) fine-tuning, a method that allows it to better manage step-by-step reasoning processes by explicitly tracing its thought patterns. This approach helps the model solve problems by making the solution process transparent and systematic. In addition, Monte Carlo Tree Search (MCTS) is employed to explore multiple reasoning paths by assigning confidence scores to alternative tokens during the problem-solving process. This technique guides Marco-o1 towards the optimal solution by selecting the most promising reasoning chain. Furthermore, Marco-o1 incorporates a reasoning action strategy that dynamically varies the granularity of actions taken during problem-solving, optimizing search efficiency and accuracy. This combination of strategies ensures that Marco-o1 is capable of dealing with both structured tasks and nuanced, open-ended challenges.

Marco-o1 addresses the limitations seen in other reasoning models by integrating a reflection mechanism that prompts the model to self-critique its solutions. By incorporating phrases that encourage self-reflection, the model is prompted to re-evaluate and refine its thought process, which improves its accuracy on complex problems. Results from the MGSM dataset demonstrate Marco-o1’s strengths: the model showed a 6.17% improvement in accuracy on the MGSM (English) dataset and a 5.60% improvement on the MGSM (Chinese) dataset compared to earlier versions. Additionally, Marco-o1 demonstrated notable results in translation tasks, such as accurately translating colloquial expressions in ways that reflect cultural nuances. This ability to handle both structured problem-solving and the subtleties of natural language highlights the practical advancement that Marco-o1 represents for AI research and application.

Conclusion
Marco-o1 represents a meaningful advancement in AI reasoning, particularly for open-ended and complex real-world problems. By leveraging techniques like Chain-of-Thought fine-tuning, Monte Carlo Tree Search, and a reasoning action strategy, Marco-o1 has demonstrated improvements over existing models, both in structured datasets and more ambiguous translation tasks. Moving forward, Alibaba plans to refine Marco-o1 by enhancing its reward mechanisms with Outcome and Process Reward Modeling, aiming to reduce randomness in its decision-making process. This will enable Marco-o1 to solve a broader range of problems more reliably and with greater accuracy.
Check out the paper, model on Hugging Face, and code repository on GitHub. All credit for this research goes to the researchers of this project. Also, don’t forget to follow us on Twitter and join our Telegram Channel and LinkedIn Group. If you like our work, you will love our newsletter.. Don’t Forget to join our 55k+ ML SubReddit.
[FREE AI VIRTUAL CONFERENCE] SmallCon: Free Virtual GenAI Conference ft. Meta, Mistral, Salesforce, Harvey AI & more. Join us on Dec 11th for this free virtual event to learn what it takes to build big with small models from AI trailblazers like Meta, Mistral AI, Salesforce, Harvey AI, Upstage, Nubank, Nvidia, Hugging Face, and more.
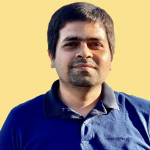
Asif Razzaq is the CEO of Marktechpost Media Inc.. As a visionary entrepreneur and engineer, Asif is committed to harnessing the potential of Artificial Intelligence for social good. His most recent endeavor is the launch of an Artificial Intelligence Media Platform, Marktechpost, which stands out for its in-depth coverage of machine learning and deep learning news that is both technically sound and easily understandable by a wide audience. The platform boasts of over 2 million monthly views, illustrating its popularity among audiences.
Be the first to comment