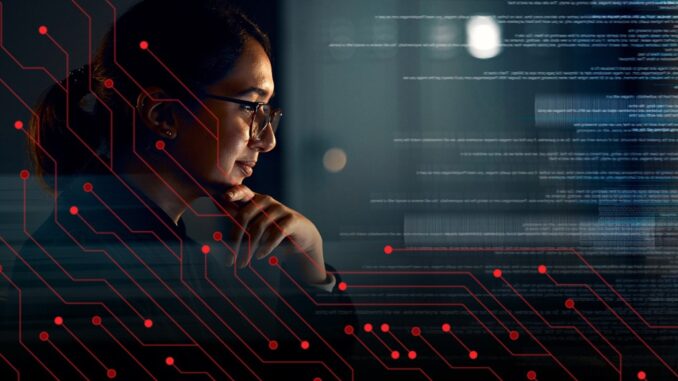
Presented by AMD
This article is part of a VB Special Issue called “Fit for Purpose: Tailoring AI Infrastructure.” Catch all the other stories here.
It’s hard to think of any enterprise technology having a greater impact on business today than artificial intelligence (AI), with use cases including automating processes, customizing user experiences, and gaining insights from massive amounts of data.
As a result, there is a realization that AI has become a core differentiator that needs to be built into every organization’s strategy. Some were surprised when Google announced in 2016 that they would be a mobile-first company, recognizing that mobile devices had become the dominant user platform. Today, some companies call themselves ‘AI first,’ acknowledging that their networking and infrastructure must be engineered to support AI above all else.
Failing to address the challenges of supporting AI workloads has become a significant business risk, with laggards set to be left trailing AI-first competitors who are using AI to drive growth and speed towards a leadership position in the marketplace.
However, adopting AI has pros and cons. AI-based applications create a platform for businesses to drive revenue and market share, for example by enabling efficiency and productivity improvements through automation. But the transformation can be difficult to achieve. AI workloads require massive processing power and significant storage capacity, putting strain on already complex and stretched enterprise computing infrastructures.
>>Don’t miss our special issue: Fit for Purpose: Tailoring AI Infrastructure.<<
In addition to centralized data center resources, most AI deployments have multiple touchpoints across user devices including desktops, laptops, phones and tablets. AI is increasingly being used on edge and endpoint devices, enabling data to be collected and analyzed close to the source, for greater processing speed and reliability. For IT teams, a large part of the AI discussion is about infrastructure cost and location. Do they have enough processing power and data storage? Are their AI solutions located where they run best — at on-premises data centers or, increasingly, in the cloud or at the edge?
How enterprises can succeed at AI
If you want to become an AI-first organization, then one of the biggest challenges is building the specialized infrastructure that this requires. Few organizations have the time or money to build massive new data centers to support power-hungry AI applications.
The reality for most businesses is that they will have to determine a way to adapt and modernize their data centers to support an AI-first mentality.
But where do you start? In the early days of cloud computing, cloud service providers (CSPs) offered simple, scalable compute and storage — CSPs were considered a simple deployment path for undifferentiated business workloads. Today, the landscape is dramatically different, with new AI-centric CSPs offering cloud solutions specifically designed for AI workloads and, increasingly, hybrid AI setups that span on-premises IT and cloud services.
AI is a complex proposition and there’s no one-size-fits-all solution. It can be difficult to know what to do. For many organizations, help comes from their strategic technology partners who understand AI and can advise them on how to create and deliver AI applications that meet their specific objectives — and will help them grow their businesses.
With data centers, often a significant part of an AI application, a key element of any strategic partner’s role is enabling data center modernization. One example is the rise in servers and processors specifically designed for AI. By adopting specific AI-focused data center technologies, it’s possible to deliver significantly more compute power through fewer processors, servers, and racks, enabling you to reduce the data center footprint required by your AI applications. This can increase energy efficiency and also reduce the total cost of investment (TCO) for your AI projects.
A strategic partner can also advise you on graphics processing unit (GPU) platforms. GPU efficiency is key to AI success, particularly for training AI models, real-time processing or decision-making. Simply adding GPUs won’t overcome processing bottlenecks. With a well implemented, AI-specific GPU platform, you can optimize for the specific AI projects you need to run and spend only on the resources this requires. This improves your return on investment (ROI), as well as the cost-effectiveness (and energy efficiency) of your data center resources.
Similarly, a good partner can help you identify which AI workloads truly require GPU-acceleration, and which have greater cost effectiveness when running on CPU-only infrastructure. For example, AI Inference workloads are best deployed on CPUs when model sizes are smaller or when AI is a smaller percentage of the overall server workload mix. This is an important consideration when planning an AI strategy because GPU accelerators, while often critical for training and large model deployment, can be costly to obtain and operate.
Data center networking is also critical for delivering the scale of processing that AI applications require. An experienced technology partner can give you advice about networking options at all levels (including rack, pod and campus) as well as helping you to understand the balance and trade-off between different proprietary and industry-standard technologies.
What to look for in your partnerships
Your strategic partner for your journey to an AI-first infrastructure must combine expertise with an advanced portfolio of AI solutions designed for the cloud and on-premises data centers, user devices, edge and endpoints.
AMD, for example, is helping organizations to leverage AI in their existing data centers. AMD EPYC(TM) processors can drive rack-level consolidation, enabling enterprises to run the same workloads on fewer servers, CPU AI performance for small and mixed AI workloads, and improved GPU performance, supporting advanced GPU accelerators and minimize computing bottlenecks. Through consolidation with AMD EPYC™ processors data center space and power can be freed to enable deployment of AI-specialized servers.
The increase in demand for AI application support across the business is putting pressure on aging infrastructure. To deliver secure and reliable AI-first solutions, it’s important to have the right technology across your IT landscape, from data center through to user and endpoint devices.
Enterprises should lean into new data center and server technologies to enable them to speed up their adoption of AI. They can reduce the risks through innovative yet proven technology and expertise. And with more organizations embracing an AI-first mindset, the time to get started on this journey is now.
Learn more about AMD.
Robert Hormuth is Corporate Vice President, Architecture & Strategy — Data Center Solutions Group, AMD
Sponsored articles are content produced by a company that is either paying for the post or has a business relationship with VentureBeat, and they’re always clearly marked. For more information, contact sales@venturebeat.com.
Be the first to comment